Jupyter notebooks in Visual Studio Code does not use the active virtual environment. Ask Question Asked 1 year, 6 months ago. Active 5 days ago. Viewed 10k times 11. I write Python code in Visual Studio Code and run the program from a terminal in which I have activated a virtual environment, and it works fine. However, if I create notebook. Jupyter Notebooks. Jupyter Notebooks are documents that contain a mix of live code (Python, R, Julia, JavaScript, and more), visualizations, and narrative text (Markdown). They're useful for breaking down concepts in a story telling form, where you can give some context and show the code below along with interactive visualizations. 2 days ago Jupyter notebooks are the go-to tool for data scientists. They make it easy to write and run some code, quickly see the results and then tweak and Read more on msdn.com. Microsoft Visual Studio; Software Development; Programming Languages; Programming; Source Code. In this episode, Robert is joined by Jeffrey Mew, who shows how you can natively edit Jupyter notebooks in Visual Studio Code. Jupyter (http://jupyter-notebo. In VS Code, the Python kernel (Python environment) used by Jupyter notebook can be independent of the Python environment we selected in VS Code (shown in the lower left corner of VS Code). As the output in the screenshot shows, the Python kernel of Jupyter you are using is 'Python3.8.5', but the module 'pygmt' is not installed in this environment.
-->Important
On January 15, 2021 the Azure Notebooks public preview site retired and has been replaced with integrated services from Visual Studio, Azure, and GitHub. To create new or execute notebook content, learn more about our other notebooks experiences from Microsoft.
In this quickstart, you will learn how to import a Jupyter Notebook for use in assorted Microsoft offerings.
If you have existing Jupyter Notebooks or want to start a new project, you can use them with many of Microsoft's offerings. Some options described in the sections below include:
Create an environment for notebooks
If you'd like to create an environment that matches that of the retired Azure Notebooks Preview, you can use the script file provided in GitHub.
- Navigate to the Azure Notebooks GitHub repository or directly access the environment folder.
- From a command prompt, navigate to the directory you want to use for your projects.
- Download the environment folder contents and follow the README instructions to install the Azure Notebooks package dependencies.
Use Notebooks in Visual Studio Code
VS Code is a free code editor that you can use locally or connected to remote compute. Combined with the Python extension, it offers a full environment for Python development including a rich native experience for working with Jupyter Notebooks.

If you have existing project files or would like to create a new notebook, you can use VS Code! For guidance on using VS Code with Jupyter Notebooks, see the Working with Jupyter Notebooks in Visual Studio Code and Data Science in Visual Studio Code tutorials.
You can also use the Azure Notebooks environment script with Visual Studio Code to create an environment that matches the Azure Notebooks Preview.
Use Notebooks in GitHub Codespaces
GitHub Codespaces provides cloud hosted environments where you can edit your notebooks using Visual Studio Code or in your web browser. It offers the same great Jupyter experience as VS Code, but without needing to install anything on your device. If you don’t want to set up a local environment and prefer a cloud-backed solution, then creating a codespace is a great option. To get started:
- (Optional) Gather any project files you would like to use with GitHub Codespaces.
- Create a GitHub repository for storing your notebooks.
- Add your files to the repository.
Use Notebooks with Azure Machine Learning
Azure Machine Learning provides an end-to-end machine learning platform to enable users to build and deploy models faster on Azure. Azure ML allows you to run Jupyter Notebooks on a VM or a shared cluster computing environment. If you are in need of a cloud-based solution for your ML workload with experiment tracking, dataset management, and more, we recommend Azure Machine Learning. To get started with Azure ML:
(Optional) Gather any project files you would like to use with Azure ML.
Create a Workspace in the Azure portal.
Open the Azure Studio (preview).
Using the left-side navigation bar, select Notebooks.
Click on the Upload files button and upload the project files.
For additional information about Azure ML and running Jupyter Notebooks, you can review the documentation or try the Intro to Machine Learning module on Microsoft Learn.
Use Azure Lab Services
Azure Lab Services allow educators to easily setup and provide on-demand access to preconfigured VMs for an entire classroom. If you're looking for a way to work with Jupyter Notebooks and cloud compute in a tailored classroom environment, Lab Services is a great option.

If you have existing project files or would like to create a new notebook, you can use Azure Lab Services. For guidance about setting up a lab, see Set up a lab to teach data science with Python and Jupyter Notebooks
Use GitHub
GitHub provides a free, source-control-backed way to store notebooks (and other files), share your notebooks with others, and work collaboratively. If you’re looking for a way to share your projects and collaborate with others, GitHub is a great option and can be combined with GitHub Codespaces for a great development experience. To get started with GitHub
- (Optional) Gather any project files you would like to use with GitHub.
- Create a GitHub repository for storing your notebooks.
- Add your files to the repository.
Next steps
-->In this article, you'll learn how to connect to an Azure Machine Learning compute instance using Visual Studio Code.
An Azure Machine Learning Compute Instance is a fully managed cloud-based workstation for data scientists and provides management and enterprise readiness capabilities for IT administrators.
There are two ways you can connect to a compute instance from Visual Studio Code:
- Remote compute instance. This option provides you with a full-featured development environment for building your machine learning projects.
- Remote Jupyter Notebook server. This option allows you to set a compute instance as a remote Jupyter Notebook server.
Configure a remote compute instance
To configure a remote compute instance for development, you'll need a few prerequisites.
- Azure Machine Learning Visual Studio Code extension. For more information, see the Azure Machine Learning Visual Studio Code Extension setup guide.
- Azure Machine Learning workspace. Use the Azure Machine Learning Visual Studio Code extension to create a new workspace if you don't already have one.
- Azure Machine Learning compute instance. Use the Azure Machine Learning Visual Studio Code extension to create a new compute instance if you don't have one.
Jupyter Vs Code
To connect to your remote compute instance:

Azure Machine Learning Extension
- In VS Code, launch the Azure Machine Learning extension.
- Expand the Compute instances node in your extension.
- Right-click the compute instance you want to connect to and select Connect to Compute Instance.
Command Palette
- In VS Code, open the command palette by selecting View > Command Palette.
- Enter into the text box Azure ML: Connect to Compute Instance.
- Select your subscription.
- Select your workspace.
- Select your compute instance or create a new one.

Navigate to ml.azure.com
Important
In order to connect to your remote compute instance from Visual Studio Code, make sure that the account you're logged into in Azure Machine Learning studio is the same one you use in Visual Studio Code.
Compute
- Select the Compute tab
- In the Application URI column, select VS Code for the compute instance you want to connect to.
Notebook
- Select the Notebook tab
- In the Notebook tab, select the file you want to edit.
- Select Editors > Edit in VS Code (preview).
A new window launches for your remote compute instance. When attempting to make a connection to a remote compute instance, the following tasks are taking place:
- Authorization. Some checks are performed to make sure the user attempting to make a connection is authorized to use the compute instance.
- VS Code Remote Server is installed on the compute instance.
- A WebSocket connection is established for real-time interaction.
Once the connection is established, it's persisted. A token is issued at the start of the session which gets refreshed automatically to maintain the connection with your compute instance.
After you connect to your remote compute instance, use the editor to:
- Author and manage files on your remote compute instance or file share.
- Use the VS Code integrated terminal to run commands and applications on your remote compute instance.
Configure compute instance as remote notebook server
In order to configure a compute instance as a remote Jupyter Notebook server you'll need a few prerequisites:
- Azure Machine Learning Visual Studio Code extension. For more information, see the Azure Machine Learning Visual Studio Code Extension setup guide.
- Azure Machine Learning workspace. Use the Azure Machine Learning Visual Studio Code extension to create a new workspace if you don't already have one.
Vscode New Jupyter Notebook
To connect to a compute instance:
Open a Jupyter Notebook in Visual Studio Code.
When the integrated notebook experience loads, select Jupyter Server.
Alternatively, you also use the command palette:
- Open the command palette by selecting View > Command Palette from the menu bar.
- Enter into the text box
Azure ML: Connect to Compute instance Jupyter server
.
Choose
Azure ML Compute Instances
from the list of Jupyter server options.Select your subscription from the list of subscriptions. If you have have previously configured your default Azure Machine Learning workspace, this step is skipped.
Select your workspace.
Select your compute instance from the list. If you don't have one, select Create new Azure ML Compute Instance and follow the prompts to create one.
For the changes to take effect, you have to reload Visual Studio Code.
Open a Jupyter Notebook and run a cell.
Important
You MUST run a cell in order to establish the connection.
At this point, you can continue to run cells in your Jupyter Notebook.
Tip
You can also work with Python script files (.py) containing Jupyter-like code cells. For more information, see the Visual Studio Code Python interactive documentation.
Next steps
Now that you've set up Visual Studio Code Remote, you can use a compute instance as remote compute from Visual Studio Code to interactively debug your code.
Tutorial: Train your first ML model shows how to use a compute instance with an integrated notebook.
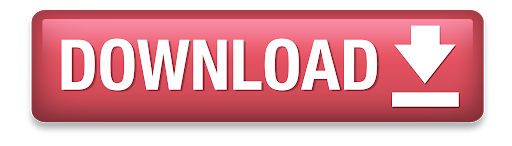